The Path to AGI is "AGI for X"
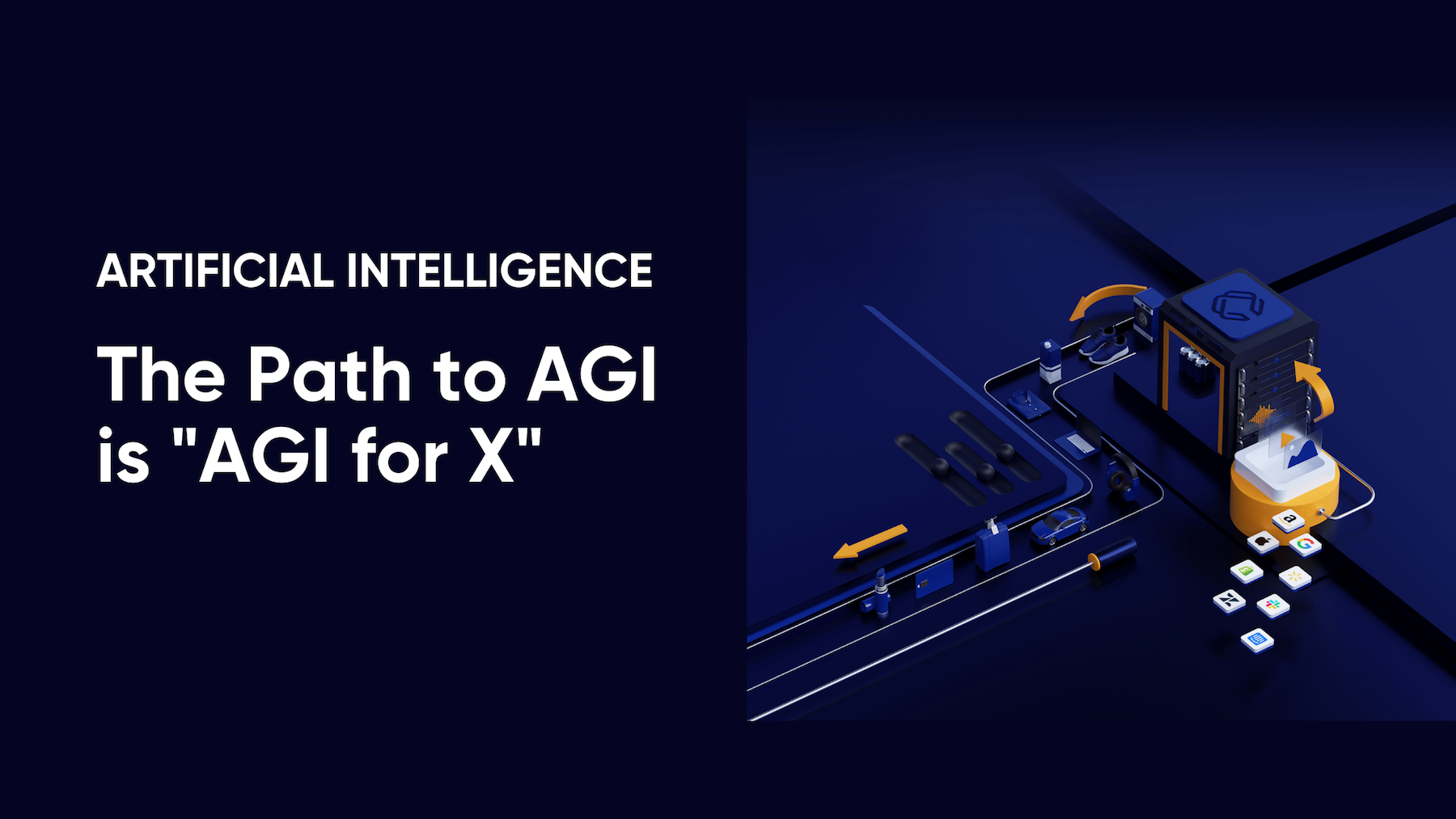
There’s a lot of discussion around Artificial General Intelligence or AGI, but not much consensus on what it takes to get there. One thing is clear: for a machine to know how to do everything, it first needs to know how to do specific things. In other words, the path to AGI is "AGI for X" — AGI for Commerce, AGI for Legal, AGI for Healthcare, and AGI for anything.

AGI for Commerce
Let’s take a look at the idea of "AGI for commerce."
Currently, AI in commerce has many diverse forms, including image classification, product tagging, sentiment analysis, recommendation engines, and so on. AGI for commerce would connect these diverse forms, with an awareness of the fabric of commerce. By learning what commerce is all about—building and selling products and services for profit—we wouldn’t need to make disparate commerce AI models.
Also, AGI for commerce would have a deep understanding of market dynamics and human behavior—important aspects when building AI-powered products or services for consumers.
Asymmetric information and big data are playing an increasing role in shaping consumer behavior, which means that businesses need to compete by understanding their customers better than they understand themselves, and building something the customer truly wants. But without a deep understanding of human nature, AI will fall short.
The Limitations of Current AI
As an example, imagine you want to build an AI-powered chatbot for customer service. You might approach the problem from two angles: on one side is technology and engineering; on the other side is psychology and behavioral economics.
Without a deep understanding of how people think and behave, your bot will be more likely to make mistakes when responding to common questions or issues customers might ask about products or services you provide them with.
Moreover, that AI model would be unable to handle any tasks outside of customer support.
With a deep understanding of human nature, however, you can build a chatbot that can handle any task or conversation. You’ll be able to add new tasks and conversations as your business grows.
The same holds true for other forms of AI in commerce. For example, recommendation engines are another important aspect of AI in commerce. Currently, there are many different types of recommendation engines: collaborative filtering, content recommender systems, and so on. But these engines don’t talk to each other; they don’t have a common language or framework for building recommendations.
If we want AI to become more useful in commerce, we need an integrated approach that takes into account all the various forms of predictive engines out there today. By learning how people think about products and services, we can create a single model that recommends products and services based on what people actually do with them.
We can also build better recommendation engines by taking into account the social aspects of consumption: how people consume together with their friends or family members; how they share recommendations with each other; how they compare products based on reviews from their peers; and so on.
Parallels With Biological Intelligence
The human brain has many diverse regions that specialize in different functions, like vision, hearing, speech, motor skills, and so on. But the brain also has a region called the hippocampus that is responsible for forming memories. This is why we can learn new things but also remember where we parked our car last week - because it’s stored in our hippocampus.
Similarly, AI systems with a deep understanding of commerce would have specialized memory banks for building market knowledge and product expertise. They would have an awareness of the fabric of commerce - from supply chains to payment systems to consumer psychology - which means they could build AI-powered products or services for consumers across various industries or verticals.
The Theory of AGI for Commerce
By learning how to learn, we could make faster progress on AGI for commerce. When you build an AI model - whether it’s for chatbots or anything else - you don’t want to build a black-box system.
A black-box system is one where the inputs and outputs are not known and cannot be verified by anyone outside of the system itself. You want to build an open-loop system so that you can verify that your model is doing what you think it should be doing with real data instead of making assumptions based on theory.
AGI Bridges
So how do we build AI models that can handle more diverse tasks without having a deep understanding of those tasks? We can start by building a bridge between different forms of AI (like visual recognition and natural language processing).
This also means bridging the vertical gap between specialized knowledge in one area and generalizable knowledge in another area. By learning from other areas, machines can gain broader expertise across multiple domains at once, which makes them smarter overall - leading to better comprehension skills when applying those new skills to specific areas like commerce.
The more ways we combine vertical knowledge, deep learning technologies, and business knowledge, the smarter our AI models become overall.
One potential solution is by using the help of knowledge graphs. Knowledge graphs are models that represent nodes (or entities) in a graph with attributes, and edges or relationships between nodes based on those attributes.
The idea is that there’s a natural link between knowledge graphs and AI models, as both can be used to define new concepts by representing them as nodes with attributes, which can then be linked together based on their relationship (i.e., type of product, demand levels).
In other words, we could, for example, use knowledge graphs to build AI models that help product teams better understand the demand of products and services in the portfolio. To achieve Artificial General Intelligence for commerce we need toolsets capable of handling multiple tasks needed across various domains simultaneously.
Commerce is a highly complex domain. There are many factors to consider when planning, analyzing, and predicting business decisions: the economics of supply and demand, the environment in which companies operate (competition, regulations, etc.), and the interests of all parties involved.
Today’s AI algorithms are designed for narrow use cases like image recognition or self-driving cars. These algorithms excel in their domain and fail when they encounter something new. A deep learning knowledge graph would allow an AI algorithm to learn from all possible sources: books, news articles, blog posts, videos, social media posts, and more.
By providing AI with a vast amount of structured data as well as unstructured text data such as blog posts or tweets, an AI could become much smarter than one that is just focused on one narrow field of expertise.
Laying the Foundations for AGI in Commerce
Let's look at how Commerce.AI is building the foundations for AGI in Commerce with its massive Product Data Engine.
The Product Data Engine is a massive knowledge database of products and services, and their attributes, which is used to provide insights for product teams. It has over 600 million products in it, and that number is growing every day as we add new sources of data. Currently, the engine sources from over 100 databases, retrieving product and service information across 56,000 categories.
Users can analyze some of the insights generated across these categories with the AI-powered market reports feature.
In other words, the Product Data Engine is a massive database of both structured and unstructured product information from a wide range of sources.
By combining this massive amount of data in the form of actionable insights and distilled knowledge, we are creating the foundation for an AGI for commerce. This is what we will need to build an AI that can think like a human. Ultimately, we’re not at AGI for commerce yet but we are getting there fast with the world’s largest product data engine.