Is Traditional Market Research Dead?
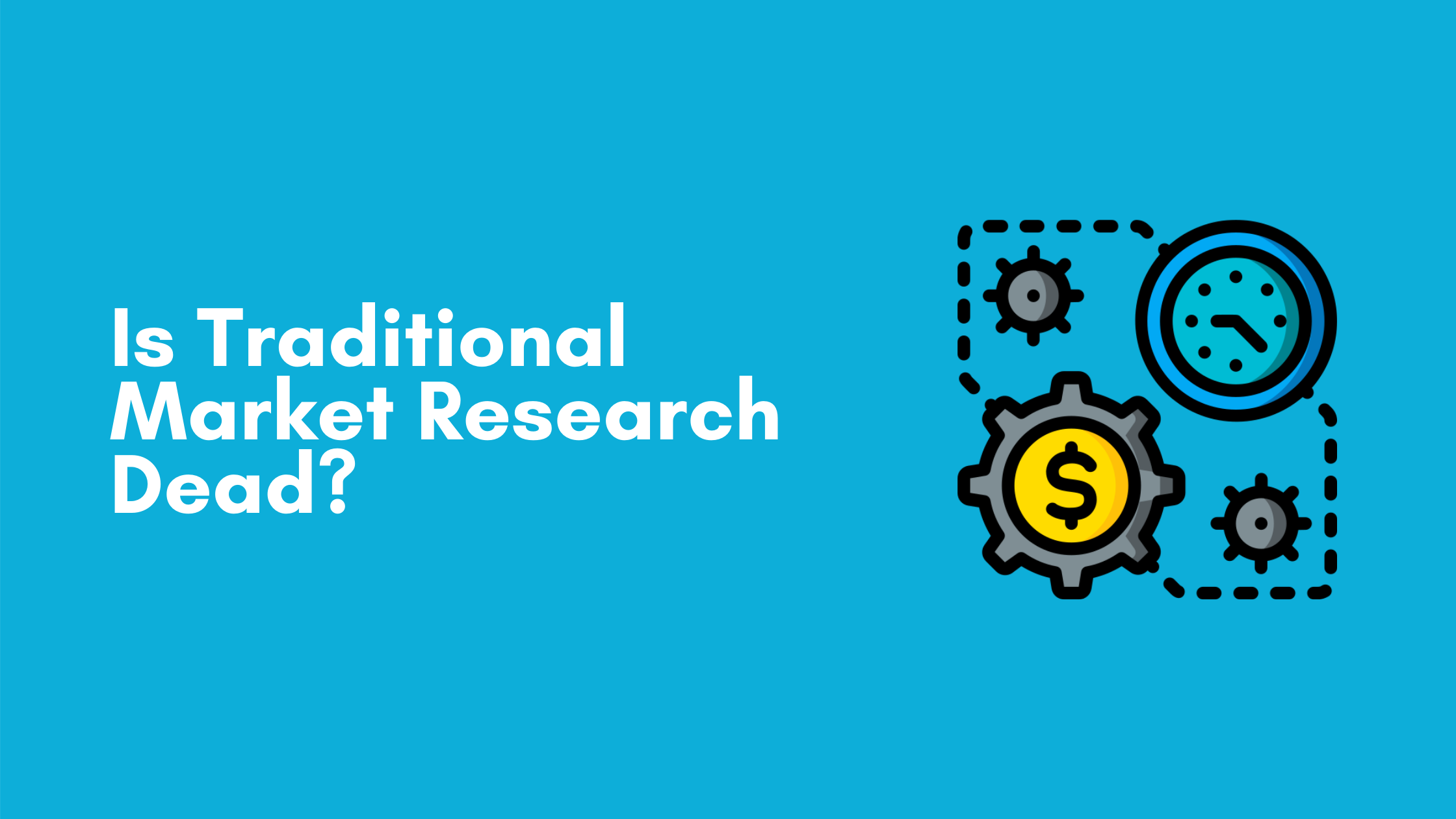
Market research is an ongoing process that involves a company or organization identifying its best customers, defining their needs, and designing creative solutions to meet those needs.
Market research usually spans six stages: data collection, data analysis, data interpretation, strategy development, formulation of marketing goals, and implementation of the marketing plan.
The stages of traditional market research
Traditional market research involves a lot of manual, tedious work. Given that there are billions of product data points available today, traditional research is only able to analyze markets at a high level, and with less accuracy than AI.
That said, traditional research still has its place in product innovation, as some insights are better than no insights.
In the first stage of traditional market research, problem identification occurs as entrepreneurs analyze their competitors' products and compare these products with their own. In the next stage - opportunity assessment - entrepreneurs determine if there is enough demand for a certain product or service in order to make production worthwhile. The third stage - customer identification - occurs when entrepreneurs list prospective customers whose interest in their product matches with their target market's desires.
These first three stages aren't enough, because they can paint too broad of a brush, and product teams need to focus on a specific target market.
Customer subtraction takes place next as entrepreneurs eliminate prospects who don't match with customer requirements according to criteria such as age range, geographic location and demographic characteristics such as occupation or family size. Evaluation follows customer subtraction, when entrepreneurs list features of potential customers that need improvement so they can better match up with target consumers' demands.
The fifth stage involves decision-making based on all previous work. In this step, teams assess costs associated with operations, versus benefits gained by having a successful product or service offering. In other words, it's time to determine profitability.
The last step in traditional market research is implementation, where marketers create a campaign strategy for launching the proposed product into the marketplace.
AI in market research
The use of AI in the market research process is a hot topic.
For product teams at companies like Suzuki and Unilever, it's a key part of their strategy to stay ahead of trends and markets.
AI automates market research by scanning over a trillion data points from unstructured data sources like Amazon, Walmart, and Target. This would be impossible to do manually or with the traditional tools that are currently being used in market research. AI doesn't just surface survey responses, but also looks at the entire spectrum of product data available online.
One of the most powerful things artificial intelligence has been able to accomplish so far is augmenting human ability by automating tasks we either can't or don't have the time for – whether that's repetitive data processing or more cognitive tasks such as marketing and analysis work.
Some other benefits of AI include quick turnaround time on insights as well as integrating quantitative analysis into qualitative insights from humans, providing better strategic context around ideas.
For example, the Commerce.AI data engine was used to generate thousands of market research reports, powered by billions of data points, which would have been impossible through traditional means.
AI is a supplement for product innovation teams
AI and machine learning are often touted as potential replacements for human work. It is true that with new technology comes new jobs, but losing jobs to automation is something every industry will eventually face.
However, AI systems are tools for product innovation teams—not replacements or substitutes for creativity and ingenuity. To apply these tools to dynamic problems requires different capabilities than simply programming a machine learning algorithm or selecting from predetermined options on an interface.
Instead of making humans obsolete, many see these technologies as giving them more time and scope to innovate because they free them from having to repeat routine tasks that machines can do quicker and better.